Practice Free DP-203 Exam Online Questions
Note: This question is part of a series of questions that present the same scenario. Each question in the series contains a unique solution that might meet the stated goals. Some question sets might have more than one correct solution, while others might not have a correct solution.
After you answer a question in this section, you will NOT be able to return to it. As a result, these questions will not appear in the review screen.
You are designing an Azure Stream Analytics solution that will analyze Twitter data.
You need to count the tweets in each 10-second window. The solution must ensure that each tweet is counted only once.
Solution: You use a hopping window that uses a hop size of 5 seconds and a window size 10 seconds.
Does this meet the goal?
- A . Yes
- B . No
B
Explanation:
Instead use a tumbling window. Tumbling windows are a series of fixed-sized, non-overlapping and contiguous time intervals.
Reference: https://docs.microsoft.com/en-us/stream-analytics-query/tumbling-window-azure-stream-analytics
You have an Azure Databricks workspace named workspace1 in the Standard pricing tier.
You need to configure workspace1 to support autoscaling all-purpose clusters.
The solution must meet the following requirements:
✑ Automatically scale down workers when the cluster is underutilized for three minutes.
✑ Minimize the time it takes to scale to the maximum number of workers.
✑ Minimize costs.
What should you do first?
- A . Enable container services for workspace1.
- B . Upgrade workspace1 to the Premium pricing tier.
- C . Set Cluster Mode to High Concurrency.
- D . Create a cluster policy in workspace1.
B
Explanation:
For clusters running Databricks Runtime 6.4 and above, optimized autoscaling is used by all-purpose clusters in the Premium plan Optimized autoscaling:
Scales up from min to max in 2 steps.
Can scale down even if the cluster is not idle by looking at shuffle file state.
Scales down based on a percentage of current nodes.
On job clusters, scales down if the cluster is underutilized over the last 40 seconds. On all-purpose clusters, scales down if the cluster is underutilized over the last 150 seconds.
The spark.databricks.aggressiveWindowDownS Spark configuration property specifies in seconds how often a cluster makes down-scaling decisions. Increasing the value causes a cluster to scale down
more slowly. The maximum value is 600.
Note: Standard autoscaling
Starts with adding 8 nodes. Thereafter, scales up exponentially, but can take many steps to reach the max. You can customize the first step by setting the spark.databricks.autoscaling.standardFirstStepUp Spark configuration property.
Scales down only when the cluster is completely idle and it has been underutilized for the last 10 minutes.
Scales down exponentially, starting with 1 node.
Reference: https://docs.databricks.com/clusters/configure.html
You have an Azure data factory.
You need to examine the pipeline failures from the last 180 flays.
What should you use?
- A . the Activity tog blade for the Data Factory resource
- B . Azure Data Factory activity runs in Azure Monitor
- C . Pipeline runs in the Azure Data Factory user experience
- D . the Resource health blade for the Data Factory resource
B
Explanation:
Data Factory stores pipeline-run data for only 45 days. Use Azure Monitor if you want to keep that data for a longer time.
Reference: https://docs.microsoft.com/en-us/azure/data-factory/monitor-using-azure-monitor
HOTSPOT
You have a Microsoft SQL Server database that uses a third normal form schema.
You plan to migrate the data in the database to a star schema in an Azure Synapse Analytics dedicated SQI pool.
You need to design the dimension tables. The solution must optimize read operations.
What should you include in the solution? to answer, select the appropriate options in the answer area. NOTE: Each correct selection is worth one point.
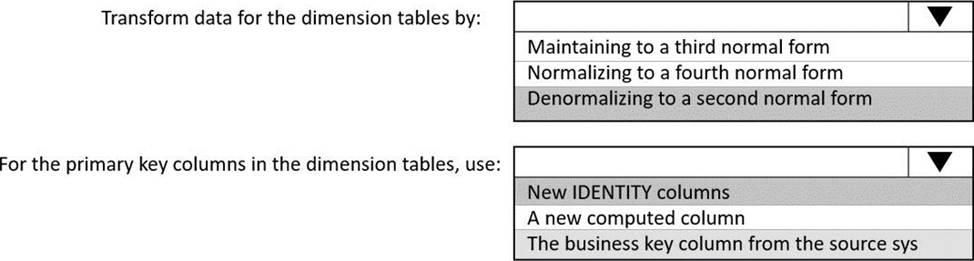
Explanation:
Box 1: Denormalize to a second normal form
Denormalization is the process of transforming higher normal forms to lower normal forms via storing the join of higher normal form relations as a base relation. Denormalization increases the performance in data retrieval at cost of bringing update anomalies to a database.
Box 2: New identity columns
The collapsing relations strategy can be used in this step to collapse classification entities into component entities to obtain flat dimension tables with single-part keys that connect directly to the fact table. The single-part key is a surrogate key generated to ensure it remains unique over time.
Example:
Note: A surrogate key on a table is a column with a unique identifier for each row. The key is not generated from the table data. Data modelers like to create surrogate keys on their tables when they design data warehouse models. You can use the IDENTITY property to achieve this goal simply and effectively without affecting load performance.
Reference:
https://www.mssqltips.com/sqlservertip/5614/explore-the-role-of-normal-forms-in-dimensional-modeling/
https://docs.microsoft.com/en-us/azure/synapse-analytics/sql-data-warehouse/sql-data-warehouse-tables-identity
You have an Azure subscription that contains the resources shown in the following table.
Diagnostic logs from ADF1 are sent to LA1. ADF1 contains a pipeline named Pipeline that copies data (torn DB1 to Dw1.
You need to perform the following actions:
• Create an action group named AG1.
• Configure an alert in ADF1 to use AG1.
In which resource group should you create AG1?
- A . RG1
- B . RG2
- C . RG3
- D . RG4
You plan to build a structured streaming solution in Azure Databricks. The solution will count new events in five-minute intervals and report only events that arrive during the interval. The output will be sent to a Delta Lake table.
Which output mode should you use?
- A . complete
- B . update
- C . append
C
Explanation:
Append Mode: Only new rows appended in the result table since the last trigger are written to external storage. This is applicable only for the queries where existing rows in the Result Table are not expected to change.
https://docs.databricks.com/getting-started/spark/streaming.html
You have an Azure Data Lake Storage account that has a virtual network service endpoint configured.
You plan to use Azure Data Factory to extract data from the Data Lake Storage account. The data will then be loaded to a data warehouse in Azure Synapse Analytics by using PolyBase.
Which authentication method should you use to access Data Lake Storage?
- A . shared access key authentication
- B . managed identity authentication
- C . account key authentication
- D . service principal authentication
B
Explanation:
Reference: https://docs.microsoft.com/en-us/azure/data-factory/connector-azure-sql-data-warehouse#use-polybase-to-load-data-into-azure-sql-data-warehouse
HOTSPOT
You have an Azure Storage account that generates 200,000 new files daily. The file names have a format of {YYYY}/{MM}/{DD}/{HH}/{CustomerID}.csv.
You need to design an Azure Data Factory solution that will load new data from the storage account to an Azure Data Lake once hourly. The solution must minimize load times and costs.
How should you configure the solution? To answer, select the appropriate options in the answer area . NOTE: Each correct selection is worth one point.
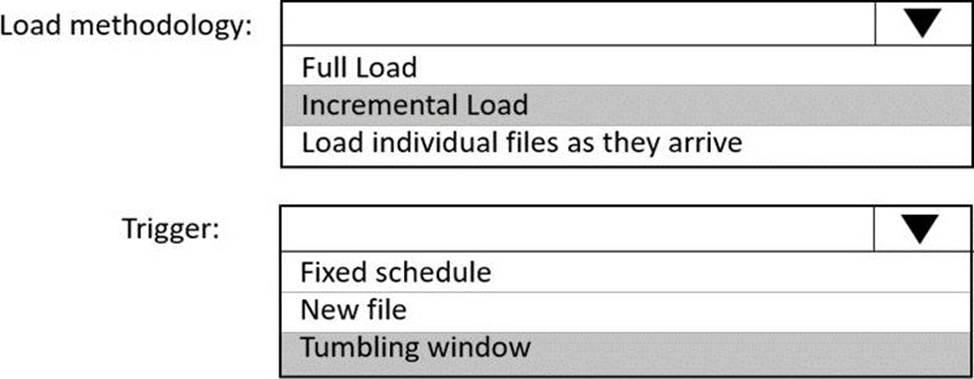
Explanation:
Box 1: Incremental load
Box 2: Tumbling window
Tumbling windows are a series of fixed-sized, non-overlapping and contiguous time intervals. The following diagram illustrates a stream with a series of events and how they are mapped into 10-second tumbling windows.
Reference: https://docs.microsoft.com/en-us/stream-analytics-query/tumbling-window-azure-stream-analytics
Note: This question is part of a series of questions that present the same scenario. Each question in the series contains a unique solution that might meet the stated goals. Some question sets might
have more than one correct solution, while others might not have a correct solution.
After you answer a question in this section, you will NOT be able to return to it. As a result, these questions will not appear in the review screen.
You plan to create an Azure Databricks workspace that has a tiered structure.
The workspace will contain the following three workloads:
✑ A workload for data engineers who will use Python and SQL.
✑ A workload for jobs that will run notebooks that use Python, Scala, and SOL.
✑ A workload that data scientists will use to perform ad hoc analysis in Scala and R.
The enterprise architecture team at your company identifies the following standards for Databricks environments:
✑ The data engineers must share a cluster.
✑ The job cluster will be managed by using a request process whereby data scientists and data engineers provide packaged notebooks for deployment to the cluster.
✑ All the data scientists must be assigned their own cluster that terminates automatically after 120 minutes of inactivity. Currently, there are three data scientists.
You need to create the Databricks clusters for the workloads.
Solution: You create a Standard cluster for each data scientist, a High Concurrency cluster for the data engineers, and a High Concurrency cluster for the jobs.
Does this meet the goal?
- A . Yes
- B . No
A
Explanation:
We need a High Concurrency cluster for the data engineers and the jobs.
Note:
Standard clusters are recommended for a single user. Standard can run workloads developed in any language:
Python, R, Scala, and SQL.
A high concurrency cluster is a managed cloud resource. The key benefits of high concurrency clusters are that they provide Apache Spark-native fine-grained sharing for maximum resource utilization and minimum query latencies.
Reference: https://docs.azuredatabricks.net/clusters/configure.html
HOTSPOT
You have an Azure Synapse serverless SQL pool.
You need to read JSON documents from a file by using the OPENROWSET function.
How should you complete the query? To answer, select the appropriate options in the answer area. NOTE: Each correct selection is worth one point.
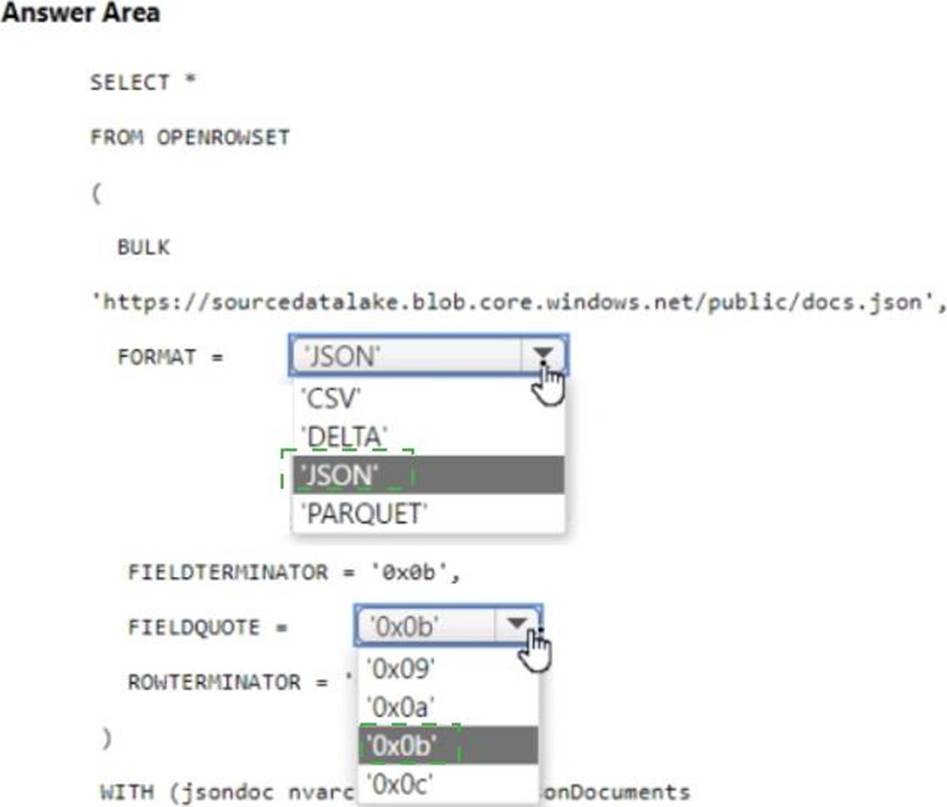